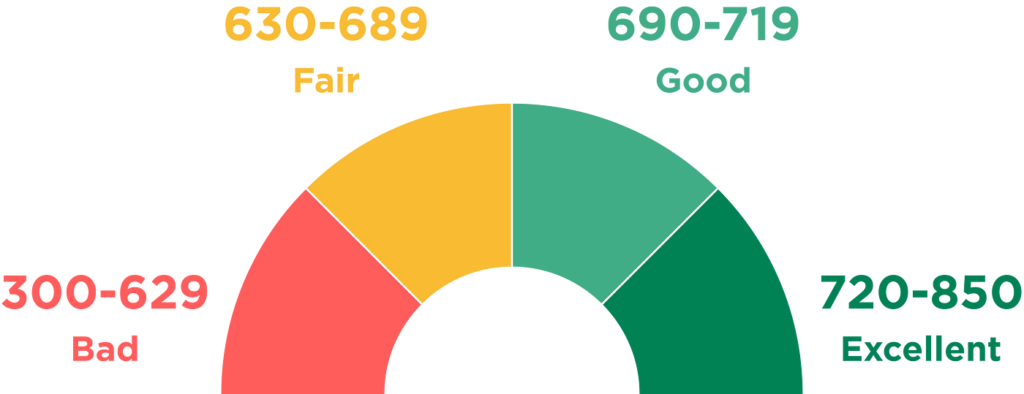
A scorecard model is a statistical tool used to assess risk, often in loan applications. It analyzes borrower data like income and credit history, assigning points to different factors. These points are totaled to create a score that predicts the likelihood of loan repayment. Scorecards help lenders make objective decisions and streamline the approval process.
Types of Scorecard Models
There are two main types of scorecard models which can be categorized based on their purpose:
1. Credit Scorecards:
Credit scorecards are used to assess the creditworthiness of borrowers. They analyze factors such as income, debt levels, and credit history to predict the likelihood of loan repayment. There are three main subcategories of credit scorecards:
- Application Scorecards: Evaluates new loan applicants and determines if they should be approved and under what terms (interest rate, credit limit).
- Behavioural Scorecards: This manages existing customer relationships, identifies opportunities for offering additional credit, and predicts potential defaults early on.
- Collection Scorecards: This is used to segment portfolios and identify risky customers.
2. Balanced Scorecards:
These are used in organizations to consider a wider range of perspectives, including customer satisfaction, internal processes, and learning and growth. There are two main types of balanced scorecards:
- Strategic Balanced Scorecard: Focuses on aligning the organization’s actions with its long-term strategy and measuring progress towards achieving those goals.
- Operational Balanced Scorecard: This helps in providing a real-time view of organization’s performance and helps identify areas for improvement.
How Scorecard Models Work in Banking Sector
Let’s delve into how scorecard models function in the banking realm.
- Data – The basis of a scorecard model is data. Banks gather a wide range of information about borrowers, which includes income, employment history, credit history, loan purpose, and even demographic details. This data acts as input for the scorecard model.
- Assigning weights – A scorecard assigns a specific weightage to each factor based on its historical correlation with loan defaults or profitability. For example, a history of missed payments might carry a higher negative weight than the borrower’s zip code.
- Statistical Technique – Statistical techniques play a vital role in this sector. A logistic regression model is used which analyzes the data, considering the weightage of each factor, and then generates a score. This score represents the borrower’s likelihood of repaying the loan (in credit scoring) or the potential success of a venture (in business lending).
- Score Interpretation – The generated score falls on a spectrum, with higher scores indicating lower risk and vice versa. Banks establish thresholds based on their risk tolerance. Borrowers who exceed the minimum score are more likely to be approved for loans under favorable terms than the ones with comparatively lower scores.
Applications of Scorecard Model
Scorecard models play a crucial role in banking for various reasons:
- Credit Risk Assessment: Scorecard models help banks assess the creditworthiness of individuals or businesses applying for loans or credit. By analyzing various factors such as credit history, income, debt-to-income ratio, and other relevant financial information, banks can determine the likelihood of a borrower defaulting on their loan.
- Decision Making: Banks can use these models to evaluate loan applications, enabling them to make consistent and reliable lending decisions.
- Risk Management: Scorecard models help banks manage their overall risk exposure. It helps to identify high-risk borrowers and adjust lending terms accordingly, such as requiring higher interest rates or collateral for riskier loans.
- Efficiency: Scorecard models streamline the loan approval process by automating much of the credit evaluation process. This improves efficiency and reduces the time and resources required to evaluate loan applications manually.
- Customization: Scorecard models can be customized to fit the specific needs and risk appetite of individual banks having types of loans or customer segments.
- Compliance: Scorecard models help banks ensure compliance with regulatory requirements by providing a systematic approach to credit risk assessment. By using standardized scoring methodologies, banks can demonstrate to regulators that they are making lending decisions in a fair and consistent manner.
- Profitability: By accurately assessing credit risk and making informed lending decisions, scorecard models improve the profitability of banks’ lending portfolios. This is done by minimizing losses from defaults and optimizing loan pricing based on risk.
Overall, scorecard models are essential tools for banks to effectively manage credit risk, make informed lending decisions, and maintain regulatory compliance. Thus, it ultimately contributes to the stability and profitability of the banking industry.
Challenges present in Scorecard Model
Scorecard models in banking, while effective in assessing credit risk, encounter several challenges:
- Data Quality and Availability: Scorecard models heavily rely on historical data for accurate predictions. Poor data quality or insufficient historical data can lead to inaccuracies in model predictions.
- Model Overfitting: Overfitting occurs when a model learns noise from the training dataset. This results in poor generalization of new data and decreased predictive performance.
- Changing Economic Conditions: Scorecard models may become less effective during economic downturns or periods of financial instability. They may not adequately capture the impact of sudden shifts in market conditions on borrower behavior.
- Emergence of New Risk Factors: Scorecard models may not capture emerging risk factors or trends that affect creditworthiness, especially in rapidly evolving industries or markets.
- Regulatory Compliance: Banks must comply with regulatory requirements when developing and deploying scorecard models. Ensuring compliance with regulations such as Basel III, IFRS9, and other local banking regulations can be challenging and requires frequent model updates.
- Model Interpretability: While scorecard models provide predictions, there is a need to understand the factors which drive these predictions. Lack of interpretability sometimes makes it difficult for the banks to explain model decisions to regulators, customers, and other stakeholders.
- Dynamic Nature of Credit Risk: Credit risk is dynamic and can change over time due to various factors such as changes in borrower behavior, market conditions, and macroeconomic factors. If scorecard models do not adapt quickly to these changes, it may lead to a decrease in predictive accuracy.
- Bias and Fairness: Scorecard models can incorporate biases in historical data, leading to unfair or discriminatory outcomes, especially towards certain demographic groups. Ensuring fairness and mitigating these biases in model predictions is crucial.
- Model Validation and Monitoring: Scorecard models require rigorous validation and ongoing monitoring to ensure they remain accurate and effective over time. This process can be resource-intensive and may require specialized expertise.
- Integration with Decision-Making Processes: Implementing scorecard models into existing decision-making processes within banks can be complex. Ensuring seamless integration and alignment with business objectives and risk appetite is essential for effective use of scorecard models.
The Future of Scorecards Models in Banking
Traditional scorecard models have long been the backbone of bank decision-making, especially in credit risk assessment. Though effective, these models sometimes lack transparency, thus making it difficult to understand why a certain score is assigned. This is where Explainable AI (XAI) steps in. XAI bridges the gap between complex AI models and human comprehension. By integrating XAI techniques, banks can leverage the power of AI for improved risk assessment while maintaining explainability. This translates to several benefits:
- Regulatory Compliance: Regulatory bodies are increasingly demanding explainability in AI-driven decisions. XAI ensures banks meet these requirements, fostering trust with regulators.
- Customer Fairness: XAI helps identify potential biases in models, preventing unfair loan denials. Customers can understand how their score is calculated, leading to greater trust and potentially improved loan applications.
- Enhanced Risk Management: By understanding the factors influencing creditworthiness, banks can make more informed decisions, potentially reducing defaults and improving overall risk management.
As Explainable AI continues to evolve, scorecards may become even more robust, transparent, and instrumental in navigating the ever-changing banking landscape.
Conclusion
The scorecard model stands as a cornerstone of modern banking practices, providing a systematic and data-driven approach to credit risk assessment and decision making. By leveraging advanced analytics and predictive modeling techniques, banks can enhance risk management, optimize resource allocation, and drive sustainable growth. However, ongoing vigilance and adaptation are imperative to address evolving market dynamics, regulatory requirements, and technological advancements, ensuring the continued relevance and effectiveness of scorecard models in an ever-changing financial landscape. The future of scorecards lies in a synergy between traditional models and XAI. This allows banks to leverage the strengths of both – the interpretability of scorecards and the predictive power of AI.