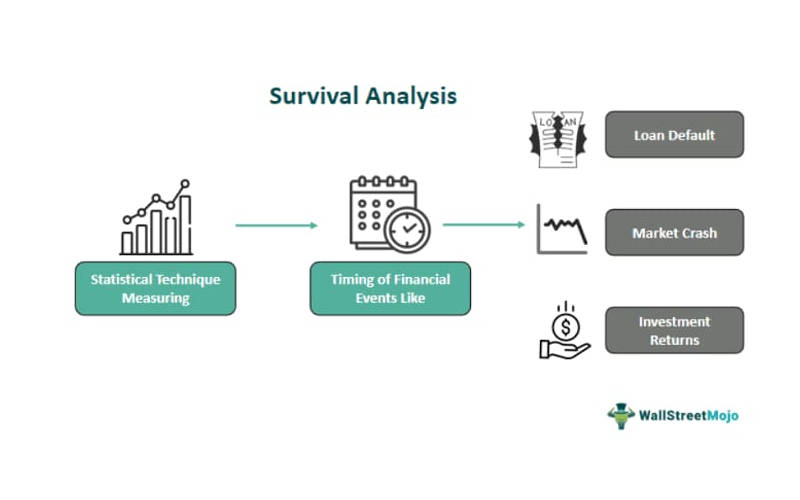
Survival analysis is a branch of statistics originally developed for analyzing time-to-event data. It has substantial application in various fields, including medicine, engineering, and economics. In recent years, survival analysis in banking has become immensely popular in this sector due to its ability to provide insights into customer behavior, risk management, and the overall financial health of institutions. This delves into the significance, methodologies, and practical applications of survival analysis in the banking industry, highlighting its potential to revolutionize how banks understand and manage their operations.
Significance of Survival Analysis in Banking
The beauty of survival analysis lies in its ability to handle censored data. This implies its accountability for situations where the event of interest hasn’t happened yet for some customers (like a loan not yet defaulted on). Traditional models would simply ignore this valuable data. By analyzing time to events, banks gain a sharper understanding of risk. They can:
- Prioritize early intervention: Identify loans at high risk of defaulting sooner, allowing for proactive steps like restructuring or collection efforts.
- Optimize resource allocation: Focus resources on customer segments most likely to churn and tailor retention strategies accordingly.
- Set more accurate risk-based pricing: Charge appropriate interest rates based on the predicted timeline of loan repayment.
Survival analysis helps banks transition from static risk assessment to a dynamic view of customer behavior. Thus, ultimately leading to better decision-making, improved profitability, and a more robust financial system.
Types of Survival Analysis
Survival analysis encompasses various statistical techniques designed to analyze time-to-event data. Key methodologies include:
- Kaplan-Meier Estimator: This non-parametric method estimates the survival function from lifetime data. It provides a stepwise function that indicates the probability of survival over time, accounting for censored data (instances where the event has not occurred by the study’s end). In banking, Kaplan-Meier estimator can be used to estimate the probability of customer retention over time.
- Cox Proportional Hazards Model: This semi-parametric model assesses the effect of several variables on the hazard or risk of an event occurring. It involves inclusion of covariates (e.g., customer demographics, account activity) to understand their impact on the survival time. In the context of loan defaults, the Cox Proportional Hazards Model can identify factors that significantly influence the likelihood of default.
- Competing Risks Analysis: In banking, multiple types of events may compete to occur, such as different types of loan defaults (e.g., early default vs. late default). Competing risks analysis allows for the modeling of such scenarios, providing a more comprehensive understanding of the factors influencing each type of event.
- Parametric Survival Models: Parametric models, like the Weibull or exponential distributions, make explicit assumptions about the functional form of the hazard rate. They estimate the time of financial events, incorporating specific assumptions about the event’s distribution.
- Censored Data Handling: It often deals with censored data, where events haven’t occurred for all observations in the dataset. Specialized techniques like the Turnbull and Nelson-Aalen estimators handle censored data and provide more accurate survival estimates.
- Accelerated Failure Time Models: These models assume that the logarithm of the survival time is linearly related to covariates. They quantify how financial events affect other factors, helping assess the impact of different variables on the timing of the event.
- Frailty Models: Frailty models help in understanding how unobserved factors may influence the timing of events like loan defaults. These models account for unobserved heterogeneity among financial instruments or individuals.
Assumptions of Survival Analysis
Survival analysis is based on certain assumptions to make meaningful predictions and inferences about the timing of financial events. Here are the key assumptions when applying it in a financial context:
- Non-Informative Censoring: Survival analysis assumes that censoring (i.e., the event of interest not occurring during the study period) is non-informative. This means that the reasons for censoring (e.g., data on a loan default being unavailable because the loan is still active) are not related to the likelihood of the event occurring.
- Constant Hazard: It assumes the hazard rate which represents the instantaneous risk of the event occurring, given that it has not occurred yet, and remains relatively constant over time.
- Independence: It is assumed that the survival times (times to financial events) of different individuals or financial instruments are independent of each other. In reality, correlations and dependencies might exist in financial markets or portfolios, but for simplicity, survival analysis often assumes independence.
- Proportional Hazards (Cox Model): When using the Cox proportional hazards model, an essential assumption is that the hazard ratio between two groups remains constant over time.
- No Competing Risks: It assumes that there is only one type of event of interest. For example, a financial instrument might be subject to both default and prepayment. In such cases, the assumption of no competing risks may not be satisfactory, and more complex models are required.
- Exogeneity: Financial events should not affect the covariates or predictors used in the survival analysis. If an event itself impacts the variables used for modeling, it can violate this assumption.
- Homogeneous Populations: It often assumes that the population under study is homogeneous, meaning that all individuals or financial instruments share the same characteristics or risk factors.
Practical Applications of Survival Analysis in Banking
Survival analysis, a statistical technique that analyzes the time-to-event data, has become an increasingly valuable tool for banks. Unlike traditional methods, it goes beyond simply predicting the likelihood of an event (like loan default) and delves into the “when” aspect. This time-sensitive approach offers significant advantages in various areas of banking, particularly risk management.
A. Credit Risk Assessment and Loan Pricing: One of the most critical applications lies in credit risk assessment. Banks use survival analysis to model the time to default (TTD) for borrowers. This allows them to:
- Predict Probability of Default (PD): By analyzing historical loan data considering factors like borrower demographics, credit history, loan characteristics, and economic conditions, models estimate the probability of default at different points in time. This provides a more nuanced picture compared to just a binary classification (default/no default).
- Set Appropriate Interest Rates: Banks can use the TTD estimates to set risk-based interest rates. Borrowers with a higher predicted risk of defaulting sooner will be charged a higher rate to compensate for the increased risk. This ensures a balance between profitability and responsible lending.
B. Portfolio Management and Capital Adequacy: Survival analysis helps banks manage loan portfolios effectively:
- Identify High-Risk Segments: By analyzing historical defaults within specific borrower categories (e.g., industry, loan type), banks can identify segments with a higher propensity for defaulting within a specific timeframe. This enables them to take proactive measures like increasing loan loss provisions or offering restructuring options.
- Regulatory Compliance: Regulations like Basel Accords require banks to maintain adequate capital reserves to absorb potential losses. Survival analysis helps banks estimate the expected loss (EL) for their loan portfolios by considering the TTD and potential severity of defaults (Loss Given Default – LGD). This ensures compliance with capital adequacy requirements.
C. Customer Relationship Management and Early Warning Systems: Survival analysis can be used to develop customer segmentation strategies:
- Identify Early Signs of Distress: By analyzing factors like missed payments, declining account balances, or changes in credit utilization, models can predict when a customer might be at risk of defaulting in the near future. This allows banks to implement early intervention strategies like personalized repayment plans or debt restructuring options.
- Retention Strategies: Conversely, the analysis can identify customers with a low risk of default. Banks can then tailor retention strategies with attractive interest rates or new product offerings for these valuable customers.
D. Operational Efficiency and Compliance: Survival analysis has applications beyond credit risk:
- Customer Churn Prediction: Banks can analyze the reason for customer account closures to identify factors leading to churn. This allows them to develop targeted marketing campaigns or improve customer service to retain valuable clients.
- Fraud Detection: Analyzing the duration between transactions can help identify fraudulent activity patterns. For example, a sudden surge in high-value transactions from a geographically unusual location might indicate potential fraud.
Survival analysis offers a powerful set of tools for the banking industry. It provides valuable insights into customer behavior, risk management, and strategic planning. It understands the timing and likelihood of key events, such as customer churn or loan defaults. In doing so, banks can make more informed decisions, improve customer retention, and mitigate risks. Advancements in technology and data analytics can further enhance the application of survival analysis in banking, driving innovation and competitive advantage in the sector.
Picture source: https://www.wallstreetmojo.com/survival-analysis/