Banks face diverse challenges to manage risk with simple spreadsheets and gut feelings. Banks choose Big Data Analytics for Risk Management
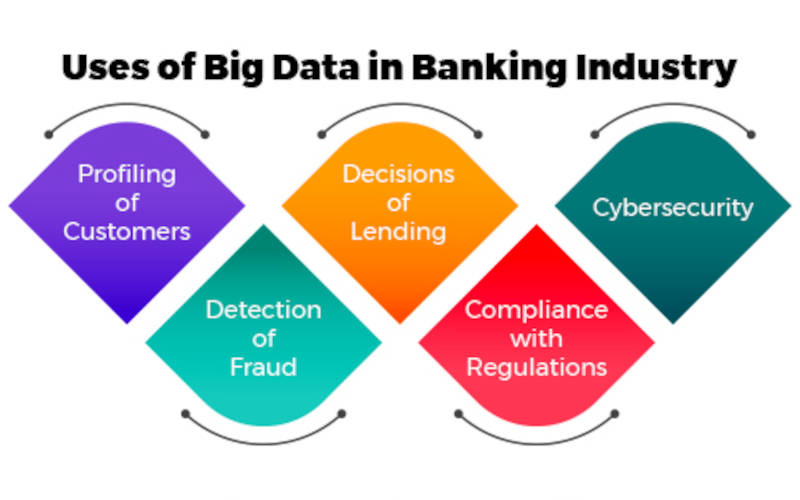
The banking industry stands at a critical juncture. Gone are the days of simple spreadsheets and gut feelings guiding risk assessments. Today, banks navigate a complex web of interconnected risks: from the ever-evolving tactics of fraudsters exploiting digital vulnerabilities to the ripple effects of global economic uncertainties and the constant pressure of tightening regulations. A single misstep can have devastating consequences, making robust risk management not just a best practice, but a necessity for survival. In this turbulent environment, banks are turning to a powerful ally: Big Data.
But what exactly is Big Data? It’s more than just a lot of data. We’re talking about massive volumes of structured and unstructured data – think customer transactions, market feeds, social media activity, regulatory filings, and even sensor data – that traditional methods struggle to process. This data deluge, however, holds immense potential. Within it lie hidden patterns, valuable insights, and predictive capabilities that are transforming industries, and banking is no exception. For financial institutions, Big Data offers a way to move beyond reactive risk management and embrace a proactive, data-driven approach.
Types of Risks Faced by Banks – A Data Driven Perspective
Before diving into how Big Data helps risk management in banking sector, it’s crucial to understand the diverse challenges banks face. These risks are not isolated; they often intertwine and amplify each other. Here’s a breakdown:
- Credit Risk: The Borrower’s Promise (and Potential Failure): This is the classic banking risk: the chance that a borrower won’t repay their loan. It’s not just about individual loans; credit risk extends to entire portfolios. Think mortgages, business loans, credit card debt – all contribute. From a data perspective, banks analyze credit scores, payment history, income, and even alternative data sources (social media sentiment, online behavior) to assess creditworthiness. The challenge lies in accurately predicting defaults, and Big Data offers the tools to go beyond traditional credit scoring.
- Market Risk: Riding the Waves of Change: Financial markets are volatile. Interest rates fluctuate, exchange rates shift, stock prices soar and plummet. Market risk is the potential for losses arising from these changes. Banks trading securities or holding large investment portfolios are particularly exposed. Big Data allows for real-time monitoring of market conditions, complex scenario analysis, and stress testing to prepare for market shocks.
- Operational Risk: The Human and System Factor: This risk comes from internal processes, human error, or system failures. Think data breaches, processing errors, or even rogue employees. Operational risk can disrupt operations, lead to financial losses, and damage reputation. Big Data can analyze internal logs, employee performance data, and even external news feeds to identify potential operational vulnerabilities and improve internal controls.
- Fraud Risk: The Ever-Evolving Threat: Fraud is a constant battle. From credit card skimming to sophisticated cyberattacks, fraudsters are always finding new ways to exploit vulnerabilities. Big Data plays a crucial role in fraud detection by identifying unusual patterns and anomalies in real-time transactions, leveraging machine learning to stay ahead of evolving fraud tactics.
- Compliance Risk: Navigating the Regulatory Maze: Banks operate in a highly regulated environment. Compliance risk is the risk of failing to meet these requirements, leading to fines, penalties, and reputational damage. Regulations cover everything from anti-money laundering (AML) to know-your-customer (KYC) rules. Big Data helps automate compliance processes, ensuring accurate data collection, analysis, and reporting, reducing the risk of regulatory breaches.
- Liquidity Risk: The Cash Flow Crunch: Liquidity risk is the risk that a bank won’t be able to meet its short-term obligations. This can happen if depositors withdraw their funds en masse or if the bank can’t easily convert assets into cash. Big Data helps banks monitor cash flow, asset values, and market conditions to maintain a healthy liquidity position.
- Reputational Risk: The Fragile Asset: While not always directly quantifiable, reputational risk can be devastating. Negative publicity, data breaches, or unethical behavior can erode public trust and lead to customer churn. Big Data can be used to monitor social media sentiment and news coverage to identify potential reputational threats and proactively address them.
How Big Data is Used for Risk Management
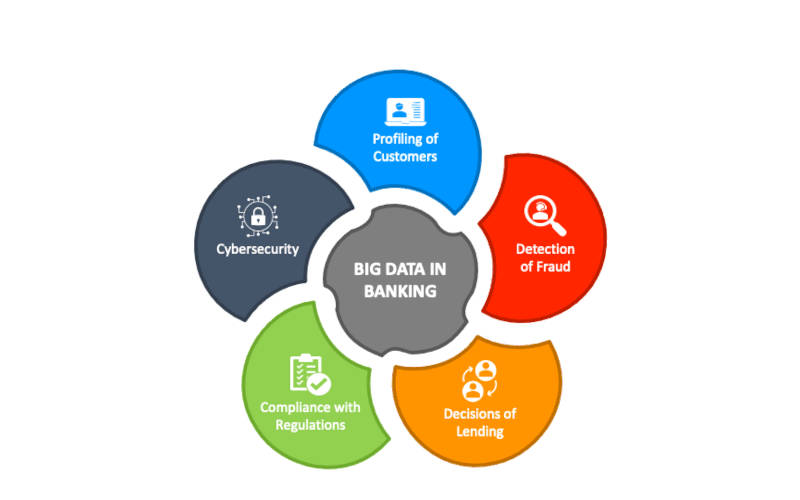
The banking industry faces a multitude of risks, ranging from credit and market risks to fraud and regulatory compliance. Traditional risk management approaches, while effective, have limitations in handling the massive volumes of data generated today. Big data analytics has revolutionized risk management by providing real-time insights, predictive capabilities, and automation, enabling banks to mitigate risks efficiently. Here’s how big data is transforming risk management in banking:
1. Credit Risk Management
Credit risk, which refers to the likelihood of borrowers defaulting on loans, is one of the most critical concerns for banks. Big data plays a crucial role in refining credit risk assessment through:
- Enhanced Credit Scoring: Traditional credit scoring models rely on limited financial history and credit bureau data. However, big data integrates diverse sources such as transaction history, social media activity, and alternative credit data (e.g., mobile payments, utility bill payments) to provide a more comprehensive risk assessment.
- Predictive Modeling for Loan Defaults: Machine learning (ML) algorithms analyze vast datasets to identify trends and predict loan default probabilities. Banks can then proactively adjust interest rates, lending terms, or decline high-risk applications.
- Personalized Risk-Based Pricing: By analyzing borrower behavior and financial patterns, banks can offer customized loan terms, ensuring that credit is extended to individuals with a higher likelihood of repayment.
2. Fraud Detection
Financial fraud, including credit card fraud, identity theft, and insider fraud, is a major concern for banks. Big data analytics significantly enhances fraud detection mechanisms:
- Real-Time Anomaly Detection: By analyzing transaction patterns across millions of accounts, big data systems can instantly flag suspicious activities, such as unusual spending behavior, geographic inconsistencies, or abnormal login attempts.
- Machine Learning for Fraud Prevention: Banks use ML algorithms, such as supervised and unsupervised learning models, to detect evolving fraud patterns. Techniques like clustering and anomaly detection help identify fraudulent transactions before they cause significant financial loss.
- Behavioral Biometrics: Advanced fraud detection systems analyze keystroke dynamics, mouse movements, and touchscreen interactions to detect unauthorized access attempts.
3. Market Risk Analysis
Market risk involves potential financial losses due to economic fluctuations, interest rate changes, and volatile stock markets. Big data helps banks navigate market risks by:
- Real-Time Market Monitoring: Advanced data analytics platforms process massive amounts of real-time market data, helping banks assess risks and adjust their portfolios accordingly.
- Sentiment Analysis: By analyzing news articles, social media discussions, and analyst reports, banks can gauge market sentiment and make informed investment decisions.
- Stress Testing and Scenario Analysis: Big data enables banks to conduct complex simulations under different economic conditions, ensuring that they can withstand market shocks and regulatory stress tests.
4. Operational Risk Management
Operational risks, such as system failures, cybersecurity threats, and human errors, can lead to financial losses and reputational damage. Big data helps mitigate these risks through:
- Employee Performance and Internal Audits: By analyzing workforce productivity, transaction logs, and workflow efficiency, banks can detect inefficiencies or potential risks before they escalate.
- Cybersecurity Risk Detection: Big data-driven security analytics continuously monitor network traffic, detect anomalies, and prevent cyberattacks, ensuring robust security infrastructure.
- Predictive Maintenance: Banks use big data to predict system failures in ATMs, online banking platforms, and IT infrastructure, minimizing downtime and operational disruptions.
5. Compliance and Regulatory Reporting
Banks must comply with stringent regulations, such as anti-money laundering (AML) and Know Your Customer (KYC) requirements. Big data simplifies compliance by:
- Automating Data Collection and Reporting: AI-driven analytics systems collect, analyze, and submit reports to regulatory authorities, reducing manual errors and ensuring compliance.
- AML and KYC Verification: Big data enables real-time customer verification by cross-referencing multiple data sources, reducing fraudulent accounts and enhancing due diligence processes.
- Regulatory Risk Monitoring: Banks leverage big data to track changes in financial regulations and adapt policies accordingly, minimizing regulatory penalties.
6. Liquidity Risk Management
Liquidity risk refers to a bank’s ability to meet its financial obligations without incurring significant losses. Big data plays a crucial role in managing liquidity risk by:
- Cash Flow Analysis: Banks use real-time analytics to monitor cash inflows and outflows, ensuring sufficient liquidity to meet short-term and long-term obligations.
- Predictive Modeling for Liquidity Needs: AI-powered forecasting models help banks anticipate future liquidity demands based on historical data and market conditions.
- Asset-Liability Management: By analyzing asset performance and liabilities, banks can optimize their portfolios and maintain a balanced liquidity position.
Tangible Benefits of Big Data-Driven Risk Management
So, how does all this Big Data analysis translate into real-world advantages for banks? The benefits are substantial and far-reaching:
- Improved Accuracy and Speed of Risk Assessments: Traditional risk assessment methods often rely on historical data and manual processes, which can be slow and inaccurate. Big Data analytics, powered by machine learning, allows banks to analyze vast amounts of data in real-time, identifying subtle patterns and correlations that humans might miss. This leads to more accurate and granular risk assessments, enabling faster and more informed decisions. Think real-time credit scoring adjustments based on up-to-the-minute transaction data.
- Proactive Risk Identification and Mitigation: Instead of reacting to risks after they materialize, Big Data enables banks to anticipate potential problems. Predictive models can forecast loan defaults, identify emerging fraud trends, and even anticipate market fluctuations. This proactive approach allows banks to take preventative measures, minimizing losses and maximizing opportunities. Imagine identifying a surge in fraudulent activity in a specific region before significant losses occur.
- Reduced Losses Due to Fraud and Defaults: By identifying fraudulent transactions in real-time and predicting loan defaults more accurately, banks can significantly reduce their financial losses. This not only improves the bottom line but also enhances customer trust and confidence. Fewer false positives in fraud detection mean less frustration for legitimate customers.
- Enhanced Regulatory Compliance: Meeting regulatory requirements can be a complex and time-consuming process. Big Data analytics simplifies compliance by automating data collection, analysis, and reporting. This reduces the risk of regulatory breaches and frees up resources for other strategic initiatives. Automated KYC/AML checks streamline onboarding and reduce compliance overhead.
- Better Decision-Making and Strategic Planning: With a more comprehensive understanding of their risk landscape, banks can make better decisions about lending, investments, and other strategic initiatives. Big Data provides the insights needed to optimize risk-return trade-offs and develop more effective business strategies. Data-driven insights inform decisions on new product development and market expansion.
- Competitive Advantage: In today’s competitive financial landscape, banks that effectively leverage Big Data for risk management gain a significant edge. They can offer more personalized products and services, make faster and more informed decisions, and minimize losses, all of which contribute to increased profitability and market share. Personalized loan offers based on individual risk profiles become a reality.
These benefits are not just theoretical. Banks that have embraced Big Data analytics for risk management are already seeing tangible results, from reduced losses to increased profitability. As Big Data technologies continue to evolve, the advantages will only become more pronounced. In the next section, we’ll look at some of the challenges banks face when implementing these powerful tools.
Challenges and Considerations in Big Data Risk Management
While the potential of Big Data in risk management is undeniable, implementing these technologies is not without its challenges. Banks must carefully consider these hurdles to ensure successful adoption and avoid costly pitfalls:
- Data Privacy and Security Concerns: Banks handle highly sensitive customer data, making privacy and security paramount. Big Data initiatives must comply with strict regulations (like GDPR, CCPA, etc.) and protect against data breaches. Robust data encryption, access controls, and anonymization techniques are essential. The risk of reputational damage from a data breach is significant, requiring meticulous planning and execution of security protocols.
- Data Quality Issues: Big Data is only as good as the data itself. Inaccurate, incomplete, or inconsistent data can lead to flawed analysis and poor decision-making. Data cleansing, validation, and standardization are critical steps. Banks need to invest in data governance frameworks to ensure data quality across all sources. Garbage in, garbage out, as they say.
- Cost of Implementing Big Data Infrastructure and Analytics Tools: Setting up the necessary infrastructure for Big Data analytics can be expensive. This includes hardware, software, and the cost of integrating different data sources. Cloud-based solutions can offer a more cost-effective alternative, but careful evaluation is essential. The ROI of Big Data initiatives needs to be clearly defined and tracked.
- Lack of Skilled Data Scientists and Analysts: Analyzing Big Data requires specialized skills in data science, machine learning, and statistical modeling. There’s a global shortage of qualified professionals, making it challenging for banks to find and retain the talent they need. Investing in training and development programs is crucial. Partnering with specialized analytics firms can also be a viable option.
- Regulatory Challenges and Evolving Compliance Requirements: The regulatory landscape is constantly evolving, and banks must keep up with new compliance requirements related to data usage and risk management. Big Data initiatives must be designed with flexibility and adaptability in mind. Collaboration with regulators and legal experts is essential to ensure compliance.
- Ethical Considerations Related to Data Usage: Using Big Data raises ethical questions about how customer data is collected, used, and shared. Banks must be transparent about their data practices and ensure that data is used responsibly and ethically. Bias in algorithms is a serious concern that needs to be addressed. Building and maintaining customer trust is paramount.
Overcoming these challenges requires a strategic approach, careful planning, and a commitment to investing in the necessary resources and expertise. By addressing these issues proactively, banks can unlock the full potential of Big Data for risk management and reap the significant benefits it offers.
Future Trends in Big Data and Banking
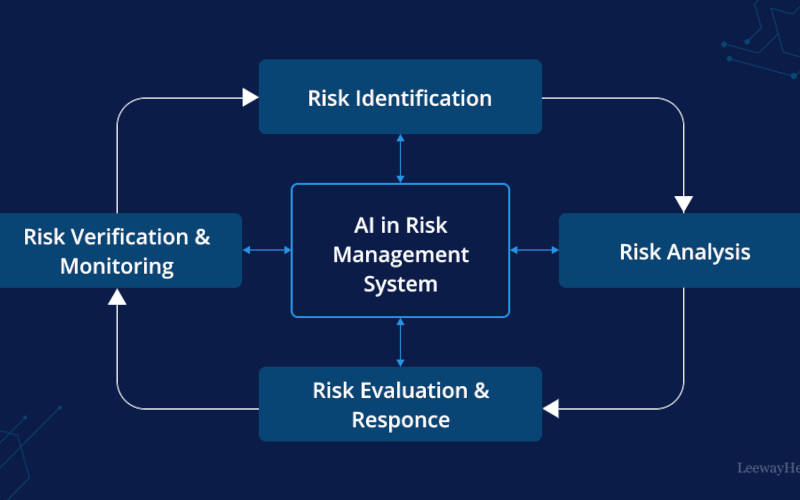
The landscape of risk management is constantly evolving, driven by technological advancements and changing market dynamics. Here’s a glimpse into the future of how Big Data will shape risk management in the banking industry:
- The Increasing Use of AI and Machine Learning in Risk Management: AI and machine learning are poised to revolutionize risk management. These technologies can automate complex tasks, identify subtle patterns, and make predictions with greater accuracy than traditional methods. Think AI-powered fraud detection systems that learn and adapt to new fraud tactics in real-time. Machine learning algorithms can be used to develop more sophisticated credit scoring models and personalize risk assessments.
- Real-Time Risk Monitoring and Predictive Analytics: The ability to monitor risks in real-time is becoming increasingly crucial. Real-time data streams, combined with advanced analytics, enable banks to identify and respond to emerging threats instantly. *Imagine a system that alerts a bank to a potential cyberattack *before* significant damage is done.* Predictive analytics will become even more sophisticated, allowing banks to anticipate future risks and take proactive measures.
- Integration of Alternative Data Sources: Traditional risk assessments often rely on limited data sources. In the future, banks will increasingly integrate alternative data sources, such as social media activity, online behavior, and even sensor data, to gain a more holistic view of risk. Consider how social media sentiment could be used to assess reputational risk or predict loan defaults. This will require robust data integration and analysis capabilities.
- The Rise of Cloud-Based Risk Management Solutions: Cloud computing offers several advantages for risk management, including scalability, cost-effectiveness, and flexibility. Cloud-based solutions enable banks to access advanced analytics tools and store vast amounts of data without investing in expensive on-premise infrastructure. Cloud-based platforms can facilitate collaboration and data sharing among different departments and even across institutions.
- Focus on Data Governance and Security: As banks rely more heavily on Big Data, data governance and security will become even more critical. Robust data governance frameworks will ensure data quality, consistency, and compliance with regulations. Blockchain technology may play a role in enhancing data security and transparency. Protecting sensitive customer data from cyberattacks will remain a top priority.
These future trends will not only enhance the effectiveness of risk management but also transform the way banks operate. By embracing these advancements, banks can better navigate the complex risk landscape, minimize losses, and unlock new opportunities for growth and innovation. The future of banking risk management is data-driven, intelligent, and proactive.
Also read: Generative AI in Banking: An Era of Financial Innovation
Embracing the Data-Driven Future of Banking Risk Management
In this post, we’ve explored how Big Data analytics is revolutionizing risk management in the banking industry. Let’s recap the key takeaways:
- Big Data empowers banks with more accurate and faster risk assessments: Moving beyond traditional methods, Big Data provides a granular and real-time view of risk, enabling more informed decision-making.
- Big Data facilitates proactive risk identification and mitigation: Predictive analytics and machine learning allow banks to anticipate potential threats and take preventative measures, minimizing losses.
- Big Data enhances regulatory compliance: Automating data collection, analysis, and reporting streamlines compliance processes and reduces the risk of regulatory breaches.
- Big Data drives better decision-making and strategic planning: By providing deeper insights into the risk landscape, Big Data empowers banks to make more informed strategic choices.
The transformative impact of Big Data on the banking industry is undeniable. It’s not just about minimizing losses; it’s about unlocking new opportunities for growth and innovation. Banks that effectively leverage Big Data for risk management gain a significant competitive advantage in today’s dynamic financial landscape. They can offer more personalized products and services, optimize risk-return trade-offs, and navigate market uncertainties with greater confidence.
Looking ahead, the future of risk management in banking is inextricably linked to Big Data. As AI and machine learning continue to advance, we can expect even more sophisticated risk assessment models and real-time monitoring capabilities. The integration of alternative data sources will provide a more holistic view of risk, while cloud-based solutions will make advanced analytics tools more accessible and scalable. However, the ethical considerations surrounding data usage, along with data privacy and security, will remain paramount and require careful attention.
The journey towards a fully data-driven approach to risk management is an ongoing process. Banks that embrace this transformation will be best positioned to thrive in the age of Big Data. What are your thoughts on the future of Big Data in banking risk management? Share your experiences and insights in the comments section below – we’d love to hear from you!