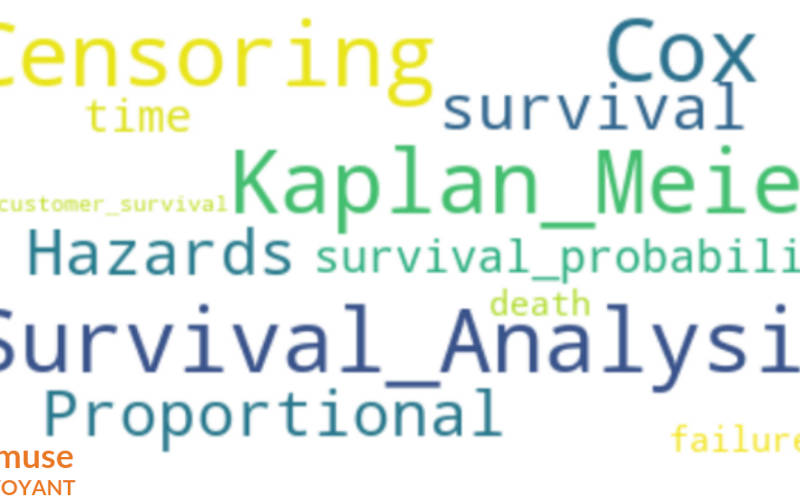
Cox Proportional Hazards Model came into effect in 1972, when David Cox developed the proportional hazards model which derives robust estimates of covariate effects using proportional hazards assumption. Proportional hazards regression is a modeling technique used to examine a times-to-event outcome, as a function of one or more exposure variables.
Understanding Cox Proportional Hazards Model
- Brief overview of survival analysis:
- What is Survival Analysis: Survival analysis is a statistical methodology specifically designed to analyze time-to-event data. In this context, “event” refers to a particular occurrence of interest, such as the occurrence of a disease, the failure of a machine, or in the banking context, a customer churning or a loan defaulting.
- Key Concepts:
- Time-to-event data: This type of data focuses on the time elapsed between a starting point (e.g., account opening, loan disbursement) and the occurrence of the event of interest.
- Censoring: Censoring arises when the exact time of the event is unknown.
- Right-censoring: The most common type, occurs when the individual is still event-free at the end of the study period.
- Left-censoring: The event of interest has already occurred before the individual entered the study.
- Interval-censoring: The event is known to have occurred within a specific time interval.
- Hazard function: The hazard function, denoted as h(t), represents the instantaneous risk of the event occurring at time ‘t’, given that the individual has survived up to that point. It provides valuable insights into the risk of the event at different time points.
- Importance in various fields: Survival analysis has broad applications across various disciplines:
- Medicine: Analyzing time to disease progression, survival rates after treatment, and the impact of different therapies.
- Engineering: Predicting the lifetime of machinery, components, and systems.
- Finance and Banking:
- Customer churn prediction: Modeling customer attrition to understand factors influencing customer lifetime value and implement retention strategies.
- Loan default prediction: Analyzing borrower characteristics and loan features to predict the probability of loan default, aiding in credit risk assessment and portfolio management.
- Credit risk assessment: Assessing the risk of credit events (e.g., bankruptcy, loan delinquency) for individual borrowers and portfolios.
- Customer lifetime value (CLTV) modeling: Estimating the expected revenue generated from a customer over their relationship with the bank.
- What is Survival Analysis: Survival analysis is a statistical methodology specifically designed to analyze time-to-event data. In this context, “event” refers to a particular occurrence of interest, such as the occurrence of a disease, the failure of a machine, or in the banking context, a customer churning or a loan defaulting.
- Introduce the Cox Proportional Hazards Model: (CPH model)
- Significance: The Cox Proportional Hazards Model is a cornerstone of survival analysis. It’s a widely used statistical model for analyzing the relationship between covariates (independent variables) and the hazard of the event of interest.
- Core Assumptions:
- Proportional Hazards Assumption: The key assumption of the Cox model. It states that the hazard ratios between any two individuals remain constant over time. In other words, the effect of a covariate on the hazard is multiplicative and does not change over time.
- Advantages:
- Flexibility: Can accommodate both continuous and categorical covariates.
- Parsimony: Doesn’t require explicit specification of the baseline hazard function, making it more flexible than parametric models.
- Widely applicable: Applicable to a wide range of survival data and has robust statistical properties.
Survival Analysis in Banking
- Key applications in banking:
- Customer churn prediction:
- Objective: To identify factors that drive customer attrition (e.g., credit card cancellations, account closures, switching to competitors).
- Benefits:
- Enables proactive customer retention strategies.
- Helps prioritize customer segments for targeted marketing and retention campaigns.
- Optimizes customer service and support to improve customer satisfaction.
- Provides insights into customer lifetime value (CLTV).
- Loan default prediction:
- Objective: To assess the likelihood of borrowers defaulting on loan repayments.
- Benefits:
- Informs credit risk assessment and lending decisions.
- Enables proactive risk mitigation measures such as loan modifications or stricter lending criteria for high-risk borrowers.
- Helps banks allocate capital more effectively and minimize loan losses.
- Credit risk assessment:
- Objective: To evaluate the overall credit risk associated with individual borrowers and loan portfolios.
- Benefits:
- Provides a more comprehensive understanding of borrower risk beyond traditional credit scoring methods.
- Helps banks comply with regulatory requirements for capital adequacy and risk management.
- Enables more accurate pricing of credit products (e.g., interest rates, loan fees).
- Customer lifetime value (CLTV) modeling:
- Objective: To estimate the total profit expected to be generated from a customer over their entire relationship with the bank.
- Benefits:
- Informs customer segmentation and prioritization strategies.
- Guides decisions on customer acquisition and retention efforts.
- Helps optimize resource allocation for customer relationship management.
- Customer churn prediction:
- Data considerations:
- Time-to-event data:
- Definition:
- Event of interest: The specific occurrence of interest, such as customer churn (cancellation of a credit card), loan default (failure to repay a loan), or bankruptcy.
- Time to event: The duration between the starting point (e.g., account opening, loan disbursement) and the occurrence of the event of interest.
- Example:
- Customer churn: Time to event is the duration between account opening and the date the customer closes their account.
- Loan default: Time to event is the duration between loan disbursement and the date of the first missed payment.
- Definition:
- Censoring:
- Right-censoring: The most common type in banking. Occurs when the customer is still active (no churn), the loan is still performing (no default), or the observation period ends before the event occurs.
- Left-censoring: Less common in banking. Occurs when the event of interest (e.g., customer churn) is known to have happened before the observation period began.
- Interval-censoring: Rare in typical banking applications. Occurs when the event is known to have happened within a specific time interval but the exact time is unknown.
- Implications: Censoring needs to be appropriately handled in the analysis to avoid biased results. Survival analysis techniques are specifically designed to account for censored observations.
- Data preparation:
- Data cleaning: Essential to ensure data quality and accuracy. Involves identifying and handling missing values, outliers, and inconsistencies.
- Feature engineering: Creating new variables from existing ones to capture relevant information. For example, creating interaction terms between variables or transforming variables to improve model performance.
- Variable selection: Choosing the most relevant predictors for the model. Techniques like forward selection, backward elimination, and regularization methods can be used to identify the most important variables.
- Time-to-event data:
Cox Proportional Hazards Model: A Deep Dive
- Model formulation:
- Core Equation: The Cox model expresses the hazard function for an individual at time ‘t’ as: h(t|x) = h0(t) * exp(β1x1 + β2x2 + … + βpxp) where:
* h(t|x) is the hazard function for an individual with covariates x1, x2, …, xp.
* h0(t) is the baseline hazard function, representing the hazard for an individual with all covariates equal to zero.
* β1, β2, …, βp are the regression coefficients associated with each covariate.
* x1, x2, …, xp are the values of the covariates for the individual.
- Hazard Ratio: The key output of the Cox model is the hazard ratio. For a one-unit increase in covariate xi, the hazard ratio is exp(βi).
- Interpretation:
- If exp(βi) > 1, the covariate xi is associated with an increased risk of the event.
- If exp(βi) < 1, the covariate xi is associated with a decreased risk of the event.
- If exp(βi) = 1, the covariate xi has no effect on the hazard.
- Interpretation:
- Proportional Hazards Assumption:
- This crucial assumption states that the hazard ratios between any two individuals remain constant over time.
- In other words, the effect of a covariate on the hazard is multiplicative and does not change over time.
- Implications: Violation of the proportional hazards assumption can lead to biased results.
- Assessing the Proportional Hazards Assumption:
- Schoenfeld residuals: These residuals assess the relationship between the residuals and time. Significant trends in the Schoenfeld residuals indicate a violation of the proportional hazards assumption.
- Graphical tests: Visual inspection of plots of scaled Schoenfeld residuals against time can help identify deviations from the proportional hazards assumption.
- Core Equation: The Cox model expresses the hazard function for an individual at time ‘t’ as: h(t|x) = h0(t) * exp(β1x1 + β2x2 + … + βpxp) where:
- Model estimation:
- Partial Likelihood Method: The Cox model is typically estimated using the partial likelihood method. This method allows for the estimation of the regression coefficients (β1, β2, …, βp) without explicitly specifying the baseline hazard function (h0(t)).
- Software Implementations: The Cox model is readily available in various statistical software packages:
- R: Packages like
survival
,survminer
provide functions for fitting the Cox model, assessing model fit, and visualizing results. - Python: Libraries like
lifelines
,scikit-survival
offer implementations of the Cox model and related functions. - SAS: Procedures like
PHREG
provide tools for fitting and interpreting Cox models.
- R: Packages like
- Model interpretation:
- Interpreting Coefficients and Hazard Ratios: As discussed earlier, the coefficients (βi) are used to calculate hazard ratios. These ratios provide insights into the relative risk associated with different covariate values.
- Identifying Significant Predictors: Statistical tests (e.g., Wald tests, likelihood ratio tests) are used to determine the statistical significance of each covariate in the model. This helps identify the most important predictors of the event of interest.
- Creating Risk Scores: Based on the estimated coefficients, risk scores can be calculated for individual subjects. These scores can be used to rank individuals based on their predicted risk of the event and to prioritize interventions.
Conclusion
- Recap of key takeaways:
- Survival analysis is a crucial tool for analyzing time-to-event data in banking, with applications in customer churn prediction, loan default prediction, credit risk assessment, and customer lifetime value modeling.
- The Cox Proportional Hazards Model is a powerful and widely used statistical method for survival analysis. It allows us to model the relationship between covariates and the hazard of the event of interest.
- Key concepts in survival analysis include time-to-event data, censoring, and the hazard function.
- The Cox model relies on the proportional hazards assumption, which needs to be carefully assessed.
- The model provides valuable insights through hazard ratios, which quantify the effect of covariates on the risk of the event.
- Limitations of the Cox model:
- Proportional Hazards Assumption: The primary limitation. Violation of this assumption can lead to biased results.
- Limited Flexibility: The Cox model assumes a multiplicative effect of covariates on the hazard. It may not be suitable for situations where the effect of covariates changes over time.
- Difficulty in Handling Time-Varying Covariates: While the Cox model can accommodate time-varying covariates, its implementation can be more complex.
- Alternative Survival Models:
- Weibull Model: A parametric model that assumes a specific distribution for the survival times. It can be used when the proportional hazards assumption is violated.
- Accelerated Failure Time Models: A class of models that directly model the effect of covariates on the time to the event.
- Future directions:
- Incorporating Machine Learning Techniques:
- Integrating machine learning algorithms (e.g., random forests, support vector machines, deep learning) with survival analysis techniques.
- Developing hybrid models that combine the interpretability of the Cox model with the predictive power of machine learning.
- Time-Varying Covariates: Developing more efficient and flexible methods for handling time-varying covariates.
- Dynamic Prediction: Developing models that can update risk predictions in real-time as new information becomes available.
- Explainable AI: Developing techniques to improve the explainability of complex survival models, making it easier to understand the factors driving risk.
- Incorporating Machine Learning Techniques: