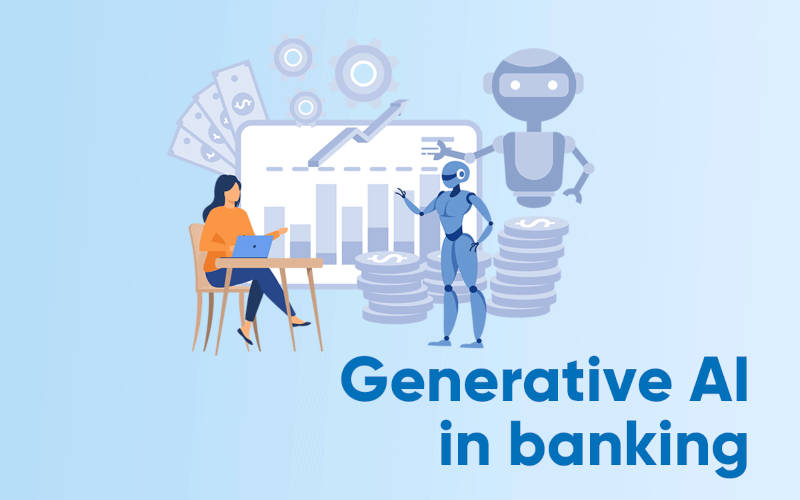
Generative AI in banking has spread in wings in the last two years as never before. It has become a game-changer not only in banking, but also in every other industry. Generative AI in banking has far-reaching impacts. While traditional machine learning and artificial intelligence have turned out to be efficient across various aspects of banking, generative AI has proven to be a game changer for the industry. GenAI is empowering financial institutions to make data-driven decisions faster, manage risks more effectively, and create innovative products that meet evolving customer needs.
Applications of Generative AI in Banking
The applications of generative AI in banking is multifaceted and it provides immense opportunities in this landscape. These applications span across customer experience, fraud detection, risk management, compliance, and operational efficiency. This article explores the diverse ways generative AI is reshaping banking.
Some of the focus areas where Gen AI leaves its mark are:
- Transaction categorization: One of the primary applications of generative AI in banking is its ability to personalize customer experiences. For instance, these systems can understand customer queries via natural language processing, analyze their financial history, and also provide personalized investment recommendations or even loan options. It can develop personalized marketing strategies too. Moreover, generative AI enhances self-service options in banking. Customers can use AI-powered platforms to draft letters for loan applications, dispute resolutions, or account upgrades. These systems dynamically adjust language and tone, ensuring the content meets regulatory and professional standards.
- Semantic Search: Using generative AI in banking helps maximizing the potential by introducing semantic search. This functionality can comprehend the context and intent behind user queries. The search process begins with query parsing. Then the customer’s inquiry is broken down into elementary entities, which are then mapped and labeled according to their roles. This helps the search engine comprehend the query by assessing the context and leveraging attributes to accurately interpret user requests.
- Pitchbook Creation: Pitchbooks are extremely critical when it comes to winning business. But it is a time-consuming affair. Junior bankers need to search through a multitude of unstructured internal and external sources, then analyze data, finally compiling it into the correct formats. Leveraging generative AI helps in gathering, processing, and summarizing information in seconds and create draft reports that are used in the final product.
- Client Onboarding: Initially, client onboarding was a tedious, paper-driven procedure. But with the advent of generative AI in banking, there has been an industrial change. It has automated document processing, providing intelligent assistance, and personalizing services. Banks can now offer superior digital onboarding which enhances lasting client relationships. In addition, usage of GenAI tools minimize human errors that occur during manual handling of complex financial information. This is taken care of by automating data entry and document processing. This helps to improve accuracy throughout the process and ensures the integrity of client data.
AI implementation also enhances operational efficiency. It ensures that customers meet all the requirements, which include KYC (know your customer), ID verifications, and automatic flagging of any missing information or potential compliance issues. Generative AI also aids in anti-money laundering (AML) efforts by analyzing transaction patterns to identify potential money laundering activities. It can simulate various laundering techniques to enhance the training of AML detection systems. Thus, making them more effective in real-world scenarios. Chatbots, or virtual assistants offer round-the-clock guidance which helps improve overall client experience and reduces the possibility of incomplete submissions. Real-time verification of client information against various databases, enhances security and reduces fraud risks.
- Personalized product recommendation: Generative AI enables banks to identify and suggest relevant products for their customers by leveraging advanced data analysis, natural language processing, and machine learning algorithms. The first step being analysis of the customer’s transaction history. The system captures the context of the transaction, taking into account factors like the payment amount, location, and the type of purchase. By drawing insights from the customer’s broader transaction history, AI can detect potential requirements that can arise from this situation. Generative AI can also analyze a customer’s existing product portfolio, risk profile, and financial goals to ensure any suggested products seamlessly integrate with the customer’s overall banking and financial plan.
- Risk management: Generative AI enhances risk assessment by enabling the generation of synthetic scenarios for stress testing and forecasting. Generative AI can help in simulation of macroeconomic scenarios, such as economic downturns or shifts in market trends, to assess their impact on a bank’s portfolio. These simulations provide insights into potential vulnerabilities and can guide decision-making. Additionally, generative AI models can generate synthetic data in order to check the robustness of risk prediction models, thus ensuring their reliability in diverse scenarios. In the credit risk management world, generative AI can aid analysis of historical loan performance and borrower behavior to predict default probabilities more accurately. It can also create hypothetical profiles of borrowers to assess how different factors, such as changes in interest rates or economic conditions, might affect repayment capabilities of customers.
- Automated Reporting: When it comes to automated reporting using generative AI, the process starts with collection of information from different sources within the bank. This includes accounting systems, transaction databases, and risk management platforms. Generative AI then analyzes this vast amount of data, employs sophisticated algorithms to identify patterns, key indicators, and tendencies that have the chances of escape during human analysis.
After the data is processed, the system creates detailed financial reports such as balance sheet data, financial results, profitability ratios, and risk analyses. The AI ensures that all regulatory requirements are met whilst presenting the information in a clear and coherent manner. Generative AI can identify future trends, look out for potential risks, and opportunities, and even provide input for financial decisions and business strategies. This automated approach significantly reduces the time and resources which used to be utilized for financial reporting. It can minimize errors associated with manual data entry and calculations. By doing so, banking professionals can focus on more strategic tasks.
Also read: Scorecard Model in Banking
Conclusion
The future of the banking industry is intertwined with the advancement of GenAI. Automation of routine tasks and facilitation of data-driven decisions allows banks to become increasingly focused on improving customer satisfaction and developing innovative financial services. GenAI helps in revolutionizing various aspects of banking, from client onboarding and transaction categorization to advanced search capabilities and personalized product recommendations. By leveraging AI capabilities, banks can offer more accurate, timely, and tailored services, meeting the evolving demands of today’s tech-savvy consumers. Besides, implementing Generative AI in banking regulatory compliance and financial reporting helps to improve efficiency and accuracy in critical back-office functions.
Image source: Techvify