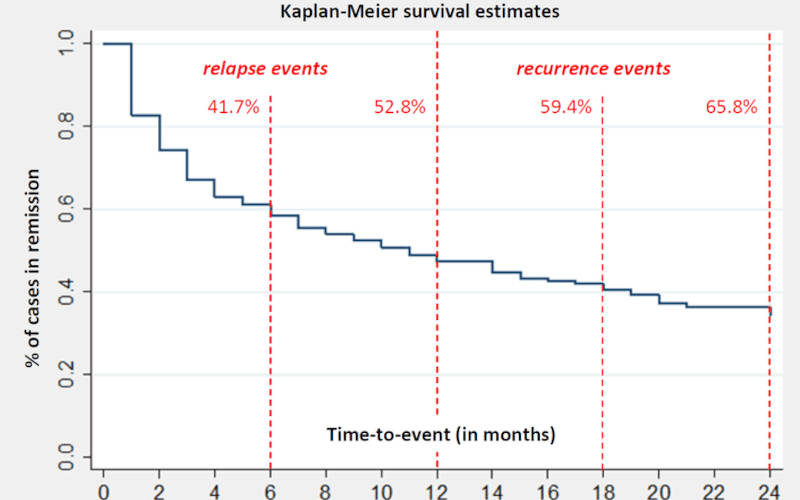
The Kaplan-Meier model, a non-parametric estimator, is one of the most widely used techniques in survival analysis, and it has found several applications in banking. From assessing loan defaults to predicting customer churn, the Kaplan-Meier estimator provides valuable insights that aid in effective decision-making. In the banking domain, understanding and predicting customer behavior, financial risks, and market trends is essential for optimizing business strategies and minimizing potential losses. Survival analysis is a statistical method designed to analyze the time until an event occurs, and it plays a crucial role in achieving this goal. This write-up explores the Kaplan-Meier model, its principles, its applications in banking, and its benefits and limitations.
Understanding the Kaplan-Meier Model
The Kaplan-Meier estimator is a statistical tool that estimates the survival function from lifetime data, primarily focusing on the probability of surviving (i.e., not experiencing a particular event) up to a certain time. Unlike parametric methods, which assume a specific distribution for the time-to-event data, the Kaplan-Meier model is non-parametric and does not require assumptions about the underlying distribution. This makes it particularly useful when dealing with data that does not follow a known distribution pattern.
The survival function represents the probability that the event of interest has not occurred by time. The Kaplan-Meier estimator computes this probability step-by-step, adjusting for the number of events (e.g., defaults, churns) and the number of individuals at risk at each time point. One of the key strengths of the Kaplan-Meier model is its ability to handle censored data, where the event of interest has not occurred for some individuals by the end of the study period.
Applications of Kaplan-Meier Model in Banking
In the banking world, the Kaplan-Meier model is primarily used to analyze customer behavior and financial risks over time. Here are some key applications of the model in the banking sector:
1. Loan Default Prediction
One of the most critical concerns for banks is the risk of loan defaults. By applying the Kaplan-Meier estimator, banks can predict the probability that a borrower will default on a loan over time. This helps financial institutions estimate the “survival” of a loan and assess the likelihood of repayment. The Kaplan-Meier model can be used to estimate the median survival time for loans, allowing banks to identify the point at which half of the loans in a given group have defaulted.
Say, if a bank wants to understand the risk of loan default based on various borrower characteristics (such as credit score, income level, loan amount, and term), the Kaplan-Meier estimator can help provide a survival curve showing how the likelihood of default changes over time. This allows the bank to refine its credit risk models and make better-informed lending decisions. Banks can also use the Kaplan-Meier estimator to compare the survival functions of different borrower segments, identifying which groups are more likely to default and adjusting their lending strategies accordingly.
2. Customer Churn and Account Closure
Customer churn is a big concern for banks. Banks strive to retain customers, and survival analysis can help predict how long customers typically stay with the bank before closing their accounts or switching to competitors. The Kaplan-Meier model can be used to estimate the time-to-churn for different customer segments, providing insights into the probability of customer retention over time. For instance, by analyzing customer data such as account type, transaction frequency, and service usage, the Kaplan-Meier estimator can generate survival curves that reveal the likelihood of account closure at different points in time.
If a bank notices that a significant portion of customers tends to leave after two years, it can use this information to implement targeted retention strategies, such as offering personalized services or loyalty programs to extend customer lifetimes. This application is particularly valuable in a highly competitive banking environment, where customer acquisition costs are high, and customer loyalty is crucial for profitability.
3. Credit Card Payment Defaults
Banks also face the challenge of predicting defaults on credit card payments. Survival analysis can help assess the time it takes for customers to default on their credit card payments. By using the Kaplan-Meier model, banks can estimate the probability that a customer will default at different time points, based on their credit history, spending patterns, and repayment behavior. For example, a bank might observe that customers who miss a payment within the first six months are at a higher risk of defaulting in the subsequent year.
The Kaplan-Meier estimator can help quantify this risk by calculating the survival probabilities of customers who have missed payments, aiding in the development of early intervention strategies to reduce the risk of default. Additionally, by examining the survival function of customers with different credit scores, the bank can assess the impact of creditworthiness on the likelihood of default.
4. Deposit and Account Activity Analysis
Banks can use survival analysis to predict how long customers are likely to maintain deposit accounts or remain engaged with the bank. The Kaplan-Meier estimator can help analyze the duration of customers’ relationships with the bank, including the likelihood of them closing their accounts or reducing their deposit activity over time. By studying various customer segments, such as those with low deposit balances or infrequent transactions, banks can identify customers who are at risk of leaving.
This allows banks to create proactive retention strategies, such as offering higher interest rates or personalized financial advice to encourage customers to maintain their accounts. Furthermore, the Kaplan-Meier model can help banks forecast the lifetime value of a customer and identify opportunities for cross-selling other products, such as loans or investment services, based on their predicted survival times.
Benefits of Kaplan-Meier in Banking
The Kaplan-Meier model offers several benefits in banking:
- Handling Censoring: The ability to handle censored data is one of the most important advantages of the Kaplan-Meier model. In banking, data can often be incomplete. This is because of some customers who have not yet defaulted, closed their accounts, or missed payments by the end of the study period. The Kaplan-Meier estimator accommodates these observations, and ensures that the analysis remains robust despite incomplete data.
- Intuitive and Easy to Interpret: The Kaplan-Meier survival curve is visually intuitive and easy for decision-makers to interpret. By observing how the survival probability changes over time, banks can make informed decisions about lending practices, customer retention, and risk management.
- No Distribution Assumptions: Unlike parametric models, which assume a specific distribution for the data (e.g., exponential or normal), the Kaplan-Meier estimator does not require such assumptions. This flexibility makes it suitable for analyzing diverse banking data with different patterns of behavior.
- Risk Stratification: The Kaplan-Meier model allows banks to segment customers based on risk factors such as credit score, loan type, and their transaction behavior. By comparing survival curves across different customer segments, banks can better understand the factors influencing customer behavior and tailor their strategies accordingly.
Limitations of Kaplan-Meier Model in Banking
Despite its strengths, the Kaplan-Meier model falls prey to some limitations in the banking context:
- Univariate Analysis: The Kaplan-Meier estimator is primarily a univariate method, which means it examines the effect of a single variable (e.g., time to default) on survival. To account for multiple variables simultaneously, more advanced methods like the Cox proportional hazards model may be needed.
- Simplistic Assumptions: This model assumes that the event of interest (e.g., loan default or account closure) occurs independently for each customer. However, in banking, customer behaviors may be influenced by external factors such as economic conditions or regulatory changes, which are not accounted for in the Kaplan-Meier model.
- Over-simplification of Complex Behaviors: While the Kaplan-Meier model provides valuable insights, it may oversimplify the complexity of customer behavior. It does not account for intricate patterns or interactions between multiple variables, such as the impact of multiple credit factors on loan default risk.
Conclusion
Thus, we see that the Kaplan-Meier model is a powerful and versatile tool for performing survival analysis in the banking domain. By estimating the probability of events such as loan defaults, customer churn, and account closures over time, the Kaplan-Meier estimator provides banks with valuable insights into customer behavior and financial risks. Even despite its limitations, the model’s ability to handle censored data, its non-parametric nature, and its intuitive interpretation make it an essential tool for banks looking to improve decision-making and optimize risk management strategies. By incorporating survival analysis into their operations, banks can enhance customer retention, refine lending practices, and minimize potential financial losses.