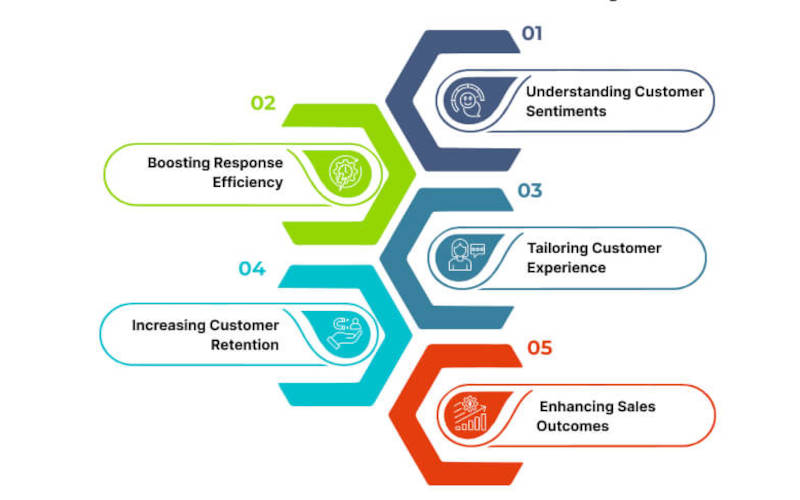
Sentiment Analysis in Banking is taking a pivotal role to improve customer experience in the face of increasing competition and digital transformation. In today’s digital era, customers express their opinions about banking services through various channels such as social media, online reviews, and customer support interactions. Understanding these sentiments is crucial for banks to enhance their services, build trust, and maintain customer loyalty.
Sentiment analysis, a branch of natural language processing (NLP) and artificial intelligence (AI), enables businesses to analyze customer emotions and opinions in real time. It helps banks gauge public perception, identify emerging trends, and respond proactively to customer concerns. The banking industry, however, faces unique challenges in sentiment analysis, including regulatory constraints, data privacy concerns, and the complexity of financial language.
Despite these challenges, sentiment analysis presents significant opportunities. It allows banks to personalize customer experiences, detect potential fraud through anomalous behavior, and improve risk management. By leveraging AI-driven insights, banks can make informed decisions, enhance customer satisfaction, and maintain a competitive edge.
Understanding Sentiment Analysis in Banking Sector
What is Sentiment Analysis?
Sentiment analysis, also known as opinion mining, is a technique used to analyze and interpret customer emotions, opinions, and attitudes from textual data. It helps businesses, including banks, understand how customers feel about their services, products, or overall brand reputation. By leveraging sentiment analysis, banks can monitor feedback from multiple channels such as social media, surveys, customer reviews, and support interactions, enabling them to make data-driven decisions to enhance customer experience.
How Does Sentiment Analysis Work?
Sentiment analysis relies on Natural Language Processing (NLP) and Machine Learning (ML) algorithms to analyze text data. NLP helps in understanding human language, breaking it down into meaningful components such as words, phrases, and sentence structures. ML models are trained on large datasets to recognize patterns in text and classify sentiments as positive, negative, or neutral. These models continuously improve over time, adapting to new language trends and contextual variations.
Different Approaches to Sentiment Analysis
- Lexicon-Based Approach – This method relies on predefined dictionaries of words associated with specific sentiments. For example, words like “excellent” and “satisfied” are positive, while “poor service” and “frustrating” are negative.
- Machine Learning-Based Approach – In this approach, ML models, such as neural networks or decision trees, are trained on labeled datasets to predict sentiment based on patterns in the data.
- Hybrid Approach – This combines both lexicon-based and machine learning techniques to improve accuracy and context understanding.
Types of Sentiment Analysis
- Polarity Detection – Determines whether a sentiment is positive, negative, or neutral.
- Emotion Detection – Identifies emotions such as happiness, frustration, trust, or anger.
- Aspect-Based Sentiment Analysis (ABSA) – Analyzes sentiment related to specific aspects, such as customer service, loan processing, or online banking experience.
Applications of Sentiment Analysis in Banking
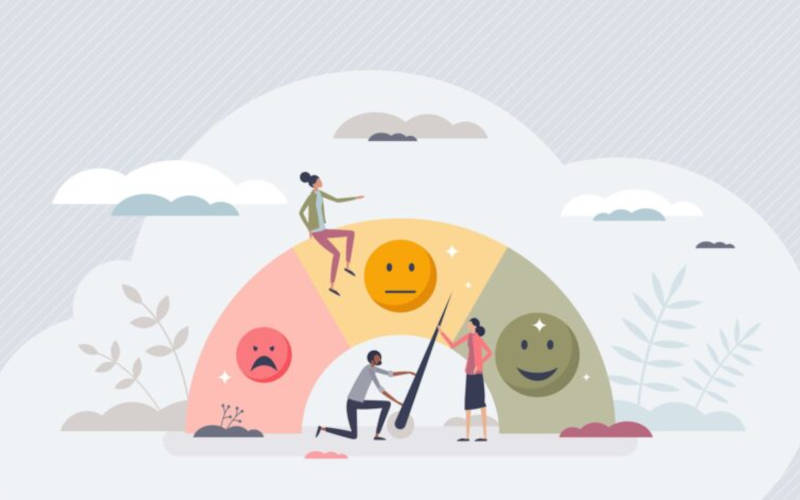
Sentiment analysis is transforming the banking sector by providing actionable insights into customer perceptions, preferences, and pain points. By leveraging artificial intelligence (AI) and natural language processing (NLP), banks can enhance customer service, develop better products, mitigate risks, refine marketing strategies, and stay ahead of competitors. Below are key applications of sentiment analysis in banking.
1. Customer Service Enhancement
Providing exceptional customer service is a priority for banks, and sentiment analysis plays a crucial role in identifying customer pain points.
- Banks can analyze customer feedback from surveys, reviews, and social media to detect common complaints, helping them improve services.
- Sentiment intensity analysis allows banks to prioritize and address urgent complaints first, ensuring a better customer experience.
- Chatbots powered by sentiment analysis can understand customer emotions and intent, leading to more effective responses and reducing frustration.
- Example: A bank notices a surge in negative sentiment regarding its online banking platform’s usability, prompting immediate interface improvements.
2. Product and Service Development
Sentiment analysis provides insights into customer preferences, helping banks refine their offerings.
- By analyzing feedback, banks can identify unmet needs and introduce new services accordingly.
- Customer sentiment helps assess the success of new product launches and identify necessary adjustments.
- Persistent negative feedback about specific features indicates areas for improvement.
- Example: A bank discovers widespread dissatisfaction with its mobile banking app’s transaction speed, leading to an optimized and faster version.
3. Risk Management and Fraud Detection
Monitoring sentiment trends can help banks mitigate risks and detect fraudulent activities.
- By analyzing social media and online forums, banks can detect discussions about fraud or security breaches and take preemptive measures.
- Anomalous sentiment shifts, such as a sudden spike in negative sentiment, can indicate a security breach or service outage.
- Market sentiment analysis helps banks anticipate financial risks and protect their reputation.
- Example: A bank identifies a rise in negative sentiment about data security concerns, prompting an immediate security audit and customer communication strategy.
4. Marketing and Customer Acquisition
Effective marketing strategies require a deep understanding of customer sentiment.
- Sentiment analysis helps banks assess how customers perceive their brand and marketing campaigns.
- By analyzing expressed needs and sentiments, banks can segment potential customers and tailor marketing efforts accordingly.
- Personalized marketing messages based on sentiment increase engagement and conversion rates.
- Example: A bank tailors its loan marketing campaigns based on customers’ financial concerns, increasing engagement and loan applications.
5. Competitive Analysis
Understanding customer sentiment about competitors, Competitive Analysis, helps banks refine their strategies.
- Banks can analyze competitor reviews and social media discussions to identify strengths and weaknesses.
- Benchmarking customer satisfaction against industry standards helps in setting improvement goals.
- Identifying competitor pain points allows banks to highlight their advantages and attract dissatisfied customers.
- Example: A bank finds that customers of a competing institution frequently complain about poor customer service. The bank then emphasizes its superior support services in marketing campaigns.
Also read: Fraud Detection in Banking Industry
Benefits of Implementing Sentiment Analysis in Banking
The adoption of sentiment analysis in the banking sector brings numerous advantages, helping financial institutions enhance customer experience, optimize operations, and stay ahead in a competitive market.
1. Improved Customer Satisfaction and Loyalty
By analyzing customer feedback in real-time, banks can identify pain points and address concerns proactively. Personalized responses and improved services lead to higher customer satisfaction and long-term loyalty.
2. Enhanced Operational Efficiency and Cost Reduction
Sentiment analysis automates the monitoring of vast amounts of customer data, reducing manual effort and operational costs. AI-driven insights enable banks to resolve issues faster, leading to better resource allocation and efficiency.
3. Better Product and Service Innovation
Understanding customer sentiments helps banks develop products and services that align with customer needs. Insights from sentiment analysis guide product improvements, ensuring a better customer experience.
4. Proactive Risk Management and Fraud Prevention
By detecting unusual sentiment trends, such as sudden negative spikes, banks can identify security threats, fraud, or compliance risks early, mitigating potential financial and reputational damage.
5. More Effective Marketing and Customer Acquisition Strategies
Sentiment analysis enables personalized marketing campaigns by understanding customer preferences. Banks can target specific segments with relevant offers, improving engagement and conversion rates.
6. Competitive Advantage in the Market
By analyzing customer sentiment towards competitors, banks can refine their strategies, highlight their strengths, and attract dissatisfied customers from rival institutions.
Also Read: Risk Management in Banking Sector using Big Data Analytics
Challenges and Considerations in Sentiment Analysis for Banking
While sentiment analysis offers significant benefits to the banking sector, implementing it comes with several challenges and considerations that must be addressed for optimal effectiveness.
1. Data Privacy and Security Concerns
Banks handle sensitive customer data, making privacy and security paramount. Ensuring compliance with data protection regulations, such as GDPR and local banking laws, is critical when analyzing customer sentiments.
2. Accuracy and Reliability Issues
Sentiment analysis tools may struggle with nuances such as sarcasm, slang, and contextual meaning. Misinterpretations can lead to incorrect insights, affecting decision-making. Continuous improvement of AI models is necessary for higher accuracy.
3. Integration with Existing Banking Systems
Implementing sentiment analysis tools requires seamless integration with existing banking infrastructure, including CRM, customer support, and risk management systems. Compatibility issues can slow down adoption.
4. Need for Skilled Personnel
Banks require data analysts and AI experts to interpret sentiment analysis insights effectively. Without skilled personnel, valuable insights may go unnoticed or misused.
5. Handling Large Volumes of Unstructured Data
Customer feedback comes from diverse sources—social media, chatbots, emails, and surveys—making it challenging to process and analyze vast amounts of unstructured data efficiently.
6. Addressing Bias in Training Data
AI models trained on biased datasets may misclassify sentiments, leading to unfair or inaccurate results. Regular model evaluation and diverse datasets help mitigate this issue.
Also Read: Generative AI in Banking – An Era of Financial Innovation
The Future of Sentiment Analysis in Banking
As technology evolves, sentiment analysis in banking is set to become even more sophisticated, offering deeper insights and enhancing customer engagement.
1. Advancements in AI and NLP
With continuous improvements in Artificial Intelligence (AI) and Natural Language Processing (NLP), sentiment analysis tools will become more accurate in understanding complex emotions, sarcasm, and contextual meanings, leading to better decision-making.
2. Integration with AI-Powered Chatbots and Personalized Banking
Banks are increasingly integrating sentiment analysis with AI-driven chatbots and personalized banking platforms. This allows chatbots to detect emotions and adjust their responses accordingly, creating a more human-like interaction.
3. Real-Time Sentiment Analysis for Immediate Service Improvements
Future banking systems will leverage real-time sentiment analysis, enabling banks to instantly address customer concerns. Automated alerts will notify support teams about negative sentiment spikes, leading to swift service interventions.
4. Predictive Sentiment Analysis for Customer Needs
Predictive analytics will help banks anticipate customer behavior, detect dissatisfaction before complaints arise, and offer proactive solutions, enhancing customer retention.
5. Ethical Considerations in Sentiment Analysis
As sentiment analysis grows, ethical concerns regarding data privacy, transparency, and bias will become more critical. Banks will need to ensure responsible AI use while maintaining customer trust.
Case Studies: Successful Implementation of Sentiment Analysis in Banking
Several leading banks have successfully integrated sentiment analysis to enhance customer experience, manage risks, and improve services.
- JPMorgan Chase uses AI-powered sentiment analysis to monitor social media and customer feedback, helping it proactively address complaints and enhance brand reputation.
- HSBC employs machine learning-based sentiment analysis to detect fraudulent activities by analyzing unusual spikes in negative sentiment related to security concerns.
- ICICI Bank integrates sentiment analysis with chatbots, enabling personalized customer interactions and quicker resolution of banking queries.
Conclusion
Sentiment analysis has become an essential tool for banks, offering valuable insights into customer perceptions, improving services, and mitigating risks. By leveraging AI and NLP, banks can enhance customer satisfaction, optimize operations, and detect fraudulent activities in real time.
From customer service improvements to risk management and competitive analysis, sentiment analysis helps banks stay ahead in an increasingly digital world. Adopting this technology is no longer optional but a necessity for financial institutions aiming to remain customer-centric and competitive.
As AI continues to evolve, sentiment analysis will play an even greater role in shaping the future of banking, making interactions more intuitive and data-driven.