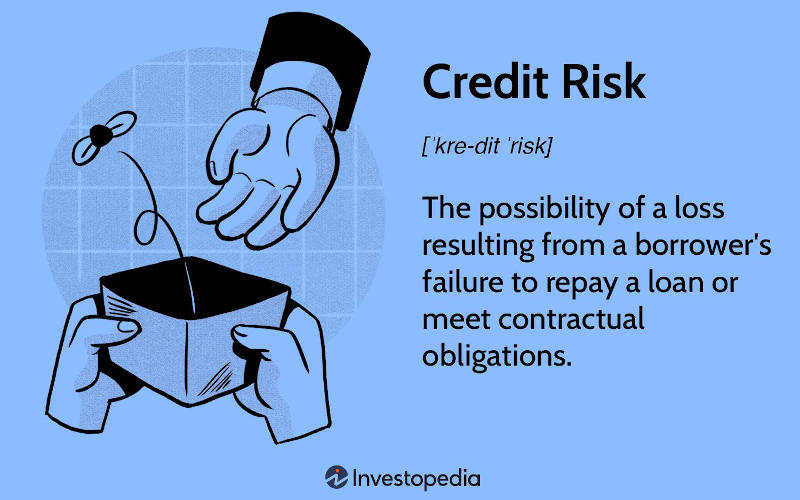
Credit risk assessment or analysis is the analysis of historical data for understanding of a borrower’s creditworthiness or assessment of the risk involved in the granting of a loan. The outcome of the analysis helps banks and financial institutions to evaluate their risks as well as their customers.
The aim of credit risk assessment is to compare the risk versus reward ratio of lending to specific individuals and companies. This helps them make informed credit decision. Some of the steps involved in it are:
- Analyzing the credit history of applicant
- Evaluating the payback capacity of the borrowed capital
- Putting into perspective the amount of capital to be borrowed
- Taking into consideration the governmental and organizational regulations
- The worth of collateral if any
Data analytics is a disruptive way in which banks can manage credit risk and minimize exposure to it.
Current challenges in Credit Risk Assessment
Some of the challenges currently faced during credit risk assessment are:
Insufficient data. Fintech companies are always in search of the correct data and getting access to it. Appropriate use of data is extremely helpful to issue loans to creditworthy customers.
Improper analysis. Manual assessment of loan application can pose harmful for potential customers. The speed at which the files are analyzed is also not very high as compared to its model-wise evaluation.
Lack of optimum tools. Improper or non-usage of the correct tools may become risky. This is because persons with a greater probability of defaults might receive the as compared to others with lower probability.
Quality and Efficiency. There is not much luxury when it comes to time. Taking more time for examination can turn the borrower to competitor. Thus, there needs to be a proper balance between high-quality credit risk assessment and the evaluation completion time. Hence, needing the use of analytics all the more.
How Analytics can improve Credit Risk Assessment
Big Data analytics helps to assess and analyze high volumes of data in minimal time. It ensures that fintech companies have all the information they need about specific customer groups as well as individuals.
Analytics for Credit Modeling. For predicting the likelihood of default, lenders leverage historical data to guess a consumer’s future behaviour. Due to this, there is less guesswork and more science involved in predicting whether someone will default or not. Earlier, credit risk models used to focus on a person’s FICO score to determine whether a consumer is a good candidate for a loan and what would be an appropriate interest rate.
Digitization of Credit Risk Modeling. In this new approach, besides customer’s credit score, real-world factors such as rent, and utility payments are taken into account. This approach sheds more light on the customer’s transactional data, assets/liabilities, deposit, or non-income information. This helps in better understanding customers who were earlier thought to be more risky.
Better way to model. Robust dataset has anonymized transactional data. Existing models can incorporate this newly introduced data. The lender finds this actions very useful. Earlier it might had been deemed as unworthy, but now it’s true value is reflected. Insight into a consumer’s assets, income and expenses opens credit markets to otherwise undeserved consumers who weren’t visible enough.
Fraud scoring models aim to optimize fraud risk control concentrating the verification on a reduced number of cases for supporting securitisation with pool audit services and IFRS 9 evaluations.
Internal Rating System (Basel Compliant) Advanced Rating systems (Both Corporate and Retail) model development from estimation, validation and review to calibration and evaluation of economic groups, including Probability of Default (PD), Loss Given Default (LGD) and Exposure at Default (EAD) models and encompassing both quantitative and judgmental approaches.
Pricing risk-based models. This is a risk-based pricing which helps to calibrate customer’s risk profile and optimize the institution’s cost structure.
Conclusion
Fintech corporations which make use of Big Data Analytics always stay ahead of the game of credit risk assessment. This is because they are never short on information about potential borrowers. Big data analytics can expand business by catering to the needs of low-risk borrowers. This is possible even if there isn’t any quantifiable credit history and credit ratings.