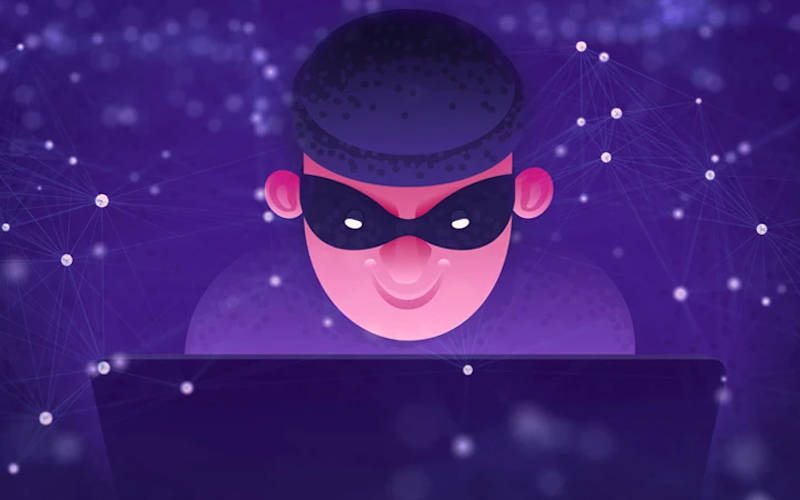
Fraud detection in banking industry is becoming crucial day by day. In this digital age, where every transaction leaves a digital trail, the banking industry faces a constant battleground: fraud. From credit card skimming to sophisticated cyberattacks, fraudsters are becoming increasingly cunning, leaving banks scrambling for solutions. But amidst this digital arms race, a powerful weapon has emerged: data science.
Traditional methods of fraud detection in banking are proving inadequate in the face of evolving tactics, making the incorporation of data science imperative. Today, data analytics is wielding a potent arsenal of algorithms and statistical models, forming an intelligent defense network against unauthorized intrusions.
Role of Data Analytics in Fraud Detection in Banking
Data Collection and Integration:
Data is the lifeline for any data science endeavor. In the case of fraud detection, diverse datasets like transaction history, user behavior, and geographical location are collected and integrated. This provides a holistic view of customer activities, enabling the identification of anomalies that may signify fraudulent behavior.
Machine Learning Algorithms:
Machine Learning algorithms are one of the key components that can play a vital role in fraud detection. These algorithms analyze historical data which helps in identifying patterns and developing models. These ML models can distinguish between legitimate and fraudulent transactions. Techniques such as supervised learning, unsupervised learning and reinforcement learning play pivotal roles in training these models.
Real-time Monitoring:
Real-time monitoring of data is of utmost importance when it comes to detecting fraud. By continuously analyzing incoming data, machine learning models are able to promptly identify and flag suspicious activities. This helps banks to respond swiftly, mitigating potential losses and safeguarding the interests of both customers and the institution.
Behavioral Analytics:
Understanding user behavior is of utmost importance when it comes to detecting anomalies. Data science leverages behavioral analytics to establish a baseline for normal user activities. Deviations from this baseline, such as an unusual transaction amount or a sudden change in spending patterns, trigger alerts for further investigation.
Predictive Modeling:
Predictive modeling is yet another key element in anticipating and preventing fraud detection in banking. By analyzing historical data, machine learning models can predict potential fraud risks.
Supervised Learning:
This technique trains algorithms on labeled data, where past fraudulent transactions are identified. The algorithm then uses this knowledge to flag similar transactions in the future.
Unsupervised Learning:
Unsupervised Learning acts as a silent observer. It maps connections and relationships between accounts and transactions. By analyzing this invisible network, algorithms can uncover hidden rings and clusters of fraudsters, thus exposing the network of criminal operations.
Deep Learning:
Inspired by the human brain, these complex neural networks can analyze vast amounts of data and identify subtle patterns that traditional methods miss. It can be thought of as a magnifying glass, revealing hidden connections between seemingly unrelated data points.
Network Analysis:
Fraudsters often operate in networks, collaborate to orchestrate complex schemes. Applying data science helps visualize these networks, identifying hidden relationships and clusters of suspicious activity.
Geospatial Analysis:
Transactions have a location, and data science can leverage this information. Imagine a thief attempting a digital bank heist from a remote island. In this case, geospatial analysis would instantly raise red flags and help in tracking down.
Also read: Geoanalytics and its usage
Real-World use cases
So far, banks have deployed multifaceted and dynamic strategies to detect and prevent fraud detection in banking, by combining human expertise with cutting-edge technologies. This has created a robust defense against evolving threats. Data science has already been making a significant impact in various areas of banking fraud detection:
- Credit Card Fraud: Machine learning algorithms analyze transaction patterns, spending habits, and location data to identify suspicious card activity in real-time. This has helped in preventing unauthorized purchases and minimizing financial losses.
- Account Takeover: By analyzing login attempts, device information, and multiple behavioral patterns, data science can successfully detect attempts to gain unauthorized access to customer accounts. This can prevent identity theft and financial losses.
- User Authentication with Biometrics: Another example is the application of biometric data into the fraud detection in banking system. By leveraging facial recognition and fingerprint scans, a bank can enhance its authentication processes, thus making it more challenging for fraudsters to gain unauthorized access to accounts.
- Money Laundering: Complex algorithms have come a long way in analyzing transaction flows and identifying suspicious patterns which might indicate money laundering activity. This helps banks comply with anti-money laundering regulations and have been able to safeguard their reputation so far.
The Future Trends in Data Analytics for Fraud Detection
The landscape of fraud is constantly evolving, and so must the tools that are used to combat it. Data science cannot afford to be a static solution; it needs to be continuously updated and refined to stay ahead of the curve. Following are some of the key trends that shape the future of fraud detection:
- Explainable AI: It is crucial to understand why complex algorithms flag certain transactions. Explainable AI algorithms can help us build a certain amount of trust and transparency when it comes to fraud detection in banking systems.
- Collaboration and Data Sharing: Sharing data and insights between banks and financial institutions can help identify and prevent cross-industry fraud. This requires secure and standardized data sharing protocols.
- Blockchain Technology: The adoption of blockchain technology holds much hope and promise for creating secure and transparent financial ecosystems. Its decentralized nature and cryptographic principles can significantly reduce the risk of fraud, providing an alternative layer of protection.
Conclusion
Data science has emerged as a game-changer when it comes to fraud detection in banking. The success of data science in fighting fraud benefits everyone. Banks will experience reduced losses and boosted customer trust. Whereas individuals will continue to enjoy greater financial security, knowing their hard-earned money is protected. It will be a win-win scenario if technology can become a shield against the cybercriminals. Banks can stay one step ahead of them, ensuring the security of financial transactions. As data science continues to evolve, we can expect even more sophisticated and effective tools which will ensure a safer and more secure banking experience for everyone.