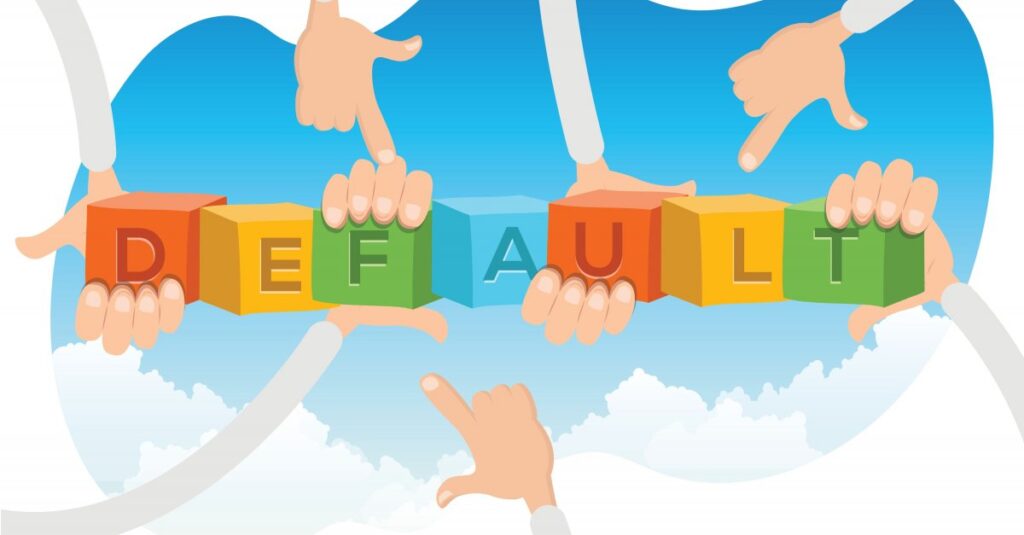
Probability of default (PD) offers a glimpse into the borrower’s future: how likely are they to miss payments and ultimately default on their debt? Understanding PD is crucial for banks and investors to individuals making personal loans. It’s the backbone of informed decision-making, helping assess risk, price loans fairly, and allocate capital wisely. When economic conditions of a country are in crisis, economic parameters need an injection of funds or additional capitals from banks to sustain their businesses, so the national economy can run even better.
Some of the macroeconomic variables which impact a country’s economy are GDP, inflation, unemployment, interest rates, etc. Probability of Default (PD) is the main component in calculating expected credit loss to assess whether there is a significant increase in credit risk and determine the probability of a debtor’s default. Probability of default (PD) estimation is essential to the calculation of expected credit loss under the Basel III framework and IFRS9.
Why is PD important?
Think of PD as a safety net. The higher the PD, the greater the chance of a borrower falling behind on payments. This translates to potential losses for lenders and a higher risk for investors. By estimating PD accurately, lenders can:
- Set fair interest rates: A borrower with a high PD needs a higher interest rate to compensate for the increased risk. PD helps ensure lenders are adequately compensated for the risk they take on.
- Make informed lending decisions: Knowing a borrower’s PD allows lenders to decide whether to offer a loan, set appropriate credit limits, and tailor loan terms to mitigate risk.
- Manage risk effectively: By accurately estimating the overall PD of their loan portfolio, lenders can calculate their expected losses and allocate capital accordingly.
Steps to calculate Probability of Default
Banks need to estimate the probability of such events occurring and set aside capital to absorb contingent losses. In accordance with the IFRS9 standard, PD estimation and development is mainly affected by a rapidly changing marketplace and a constant increase in the number of market participants. Some of the steps to calculate PD estimates are : selection of ample data for analysis, analysis of account performance (default flag) for the next twelve months based on the predefined default definitions, finally analyzing default rate analysis for each of the buckets to find out the default percentage of accounts in each bucket. This is later used as an estimation for PD in the future. Micro-segment credit and macro-segment work differently. PD for the micro-segment credit is, indeed, affected by changes in macroeconomic variables. These include GDP, inflation, exchange rate, and unemployment rate.
Supervision on macroeconomic changes by the government and regulators is very important. Once any model is developed, it must pass through validations, reviews from different segments. Internal, external testing, approval from the region, then only is it allowed for deployment.
Components of Probability of Default
In July 2014, the IFRS9 introduced an expected credit loss (ECL) framework. This framework is built to help banks recognize and manage potential credit losses for financial statement–reporting purposes. ECL model comprises macroeconomic factors like GDP growth rate. Under the Basel accord, PDs are commonly estimated as through-the-cycles to neutralize economic fluctuations and achieve lower volatility in credit risk capital requirements. However, on the contrary, under the IFRS9, PD estimates should be point-in-time and include forward-looking information, especially in case of macroeconomic forecasts. Logistic Regression is widely used in case of building PD models due to its classification technique. In the banking sector, logit models are used in the calculation of default rates. GDP is a strong predictor of default rates.
Challenges that arise
Multiple data sanity checks are done before the data is made usable. Sometimes data is not available for direct usage. It needs to be derived as per requirement. We might need to expand the time span, enhance the backtesting period, and expand the samples. More accurate results of the measurement of impacts of macroeconomic changes on PD can be achieved through this. COVID-19 had a significant impact on the robustness of the data and the different PD determinants. Sometimes binomial models might be biased for the model. Thus, some market-based index is always advisable to introduce in a PD model.